What is AI in Marketing, and What It Can (and Can’t) Do
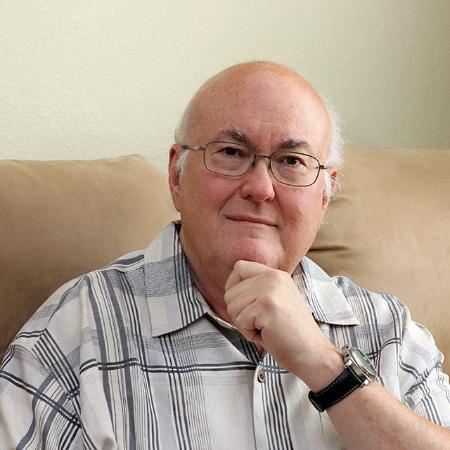
By Alan C. Brawn CTS, DSCE, DSDE, DSNE, DCME, DSSP, ISF-C
There is no place to hide from the influence that artificial intelligence (AI) has (and increasingly will have) on our lives both personal and professional. Reading what you will, it seems that AI is either the greatest boon to mankind since the invention of the wheel or the death knell of humankind as we know it. Dramatic of course, but there is a modicum of truth in both extremes. To say the least this is fodder for inquiring minds and hence the motivation to dig deeper into the facts, promise, and fallacies concerning AI… especially where it affects marketing in general, and digital signage in particular.
I took my own advice (for a change!) and decided to start my research at the very beginning, and slowly work my way forward. Time consuming for sure, but in the end well worth the effort. This resulted in the writing of an AI trilogy for Commercial Integrator magazine. The objective there was to share what I learned about the foundations of AI (in a more concise and digestible manner) to be used as a launchpad from which further learning could take place. Of course, further learning will be necessary! I started my journey with the founders of the concept and what they originally thought of AI, along with some chronologically significant dates. Don’t worry… there will be no test after this one! I followed this by demythologizing how AI actually works and ended with a treatise on the upsides and caveats surrounding AI. With this under our proverbial belts we can dig deeper for followers of the Digital Signage Federation. In proper order it is Artificial Intelligence and under that Machine Learning, and then the subset most meaningful to us in marketing and digital signage, Generative AI. Here is the story.
Artificial Intelligence
The umbrella of Artificial Intelligence refers to creating intelligent machines that mimic human-like cognitive abilities. AI-enabled programs can analyze and contextualize data to provide information or automatically trigger actions without human interference. AI encompasses a range of techniques, algorithms, and methodologies aimed at enabling computers to perform tasks that typically require human intelligence. These tasks can include natural language processing, problem-solving, pattern recognition, planning, and decision-making.
Put in context, artificial intelligence refers to the general ability of computers to emulate human thought and perform tasks in real-world environments, while machine learning refers to the technologies and algorithms that enable systems to identify patterns, make decisions, and improve themselves through experience and data.
Machine Learning
Machine learning is a type of artificial intelligence. Through machine learning, practitioners develop artificial intelligence through models that can “learn” from data patterns without human direction. The unmanageable (by humans, anyway) volume and complexity of data that is now being generated has unleashed the potential and need of machine learning.
Machine learning involves inputting a large volume of data to a machine so that it can learn and make predictions, find patterns, or classify data. The three basic machine learning types are supervised, unsupervised, and reinforcement learning.
- Supervised
- This type of machine learning feeds existing data (input and output) in machine learning algorithms, with processing in between each input/output pair that allows the algorithm to adjust the model to create outputs as closely aligned with the desired result as possible. In supervised learning, the machine is taught by example. The operator provides the machine learning algorithm with a known dataset that includes desired inputs and outputs, and the algorithm must find a method to determine how to arrive at those inputs and outputs. The algorithm makes predictions and is corrected by the operator – and this process continues until the algorithm achieves a high level of accuracy and performance.
- Unsupervised
- Unsupervised learning is a type of machine learning technique (sans a human operator) in which an algorithm discovers patterns and relationships using unlabeled data. The machine determines the correlations and relationships by analyzing available data. The algorithm tries to organize that data in some way to describe its structure. As it assesses more data, its ability to make decisions on that data gradually improves and becomes more refined. The primary goal is to discover hidden patterns, similarities, or clusters within the data, which can then be used for various purposes, such as data exploration, visualization, dimensionality reduction, and more.
- Reinforcement
- Reinforcement learning is a machine learning technique that trains software to make decisions to achieve the most optimal results. It mimics the trial-and-error learning process that humans use to achieve their goals. Software actions that work towards your goal are reinforced, while actions that detract from the goal are ignored. As data is processed, they learn from the feedback of each action and self-discover the best processing paths to achieve final outcomes. Reinforced learning is a powerful method to help AI systems achieve optimal outcomes in unseen environments.
Until recently, machine learning was largely limited to predictive models, used to observe, and classify patterns in content. Generative AI was a breakthrough with implications that we are only beginning to comprehend. Machine learning specializes in developing algorithms that learn from data to make predictions or decisions, and Generative AI leverages machine learning to generate original and realistic content.
Generative AI
Generative AI is an advanced branch of AI that utilizes machine learning techniques to generate new, original content such as images, text, audio, and video. Unlike traditional machine learning, which focuses on mapping input to output, generative models aim to produce novel and realistic outputs based on the patterns and information present in the vast amounts of training data available for input in specific algorithms. The models output new content by referring to the data they have been trained on, making new predictions. Generative AI is used in any AI algorithm or model that utilizes AI to output a brand-new attribute. Before discussing the proverbial elephant in the room of AI in marketing, let’s look at a couple of generative AI issues to consider.
Shortcomings of Generative AI
Generative AI uses a huge amount of content from across the internet as its “fuel”, and then using said information they train to make predictions and create an output based on user provided prompts, inquiries, or input. The issue (read that concern) is the quality of the data the models are fed. We have little way of knowing where the information has come from, and how the algorithms have processed data to generate content. Some generative AI models are attempting to bridge that questionable source gap by providing footnotes with sources that are intended to enable users to not only know where their response is coming from, but to also verify the accuracy of the response. Attempting is the key word here. Even if the responses sound plausible there are no guarantees the prediction/answer/output will be correct. Think garbage in and garbage out.
As one expert pointed out, “The responses might also incorporate biases inherent in the content the model has ingested from the internet, but there is often no way of knowing whether that’s the case. Both of these shortcomings have caused major concerns regarding the role of generative AI in the spread of misinformation.”
While the results from generative AI can be intriguing, helpful, and productive, it would be unwise, certainly in the short term, to totally rely on the information or content they create.
AI in Marketing from the Experts
An undeniable fact is that companies are rapidly adopting AI based solutions to promote operational efficiency with the intended consequence of improving the customer experience. Through the employment of Artificial Intelligence (AI) marketing platforms, marketers can gain a more nuanced, comprehensive understanding of their target audiences. This begs the question… what is AI Marketing?
A key role of marketing is to facilitate the transfer of information and insights from the outside environment (customers, competition) to the inside of the company and vice versa. AI has been — and increasingly will be — transformational in this area because it enables the processing of vast amounts of information and affords a degree of flexibility, analysis, targeting, and personalization (for example, in advertising) that human beings simply can’t manage on their own.
McKinsey (following their research) has unequivocally declared that “AI is poised to disrupt marketing and sales in every sector.” They predict that generative AI could increase marketing productivity by 5% to 15% across a range of applications, among them personalized content creation at scale, enhanced customer engagement, more robust customer and data insights, improved lead identification, and development.
Research showed that most marketing and sales leaders (78%) acknowledge that generative AI is likely to have “some” impact on their company — and when asked to identify its potential benefits, they most frequently picked enhanced effectiveness, employee efficiency, and customer experience. Several respondents noted that “Artificial Intelligence can eliminate many repetitive, manual [tasks], improve employees’ attention and action efficiency, such as interaction with customers, follow-up of sales prospects, and improvement of customer satisfaction.”
The marketing and sales leaders surveyed foresaw applications for generative AI that encompass content creation, enhancements in personalization, and insights generation. One early adopter said, “AI can analyze consumers’ shopping habits, preferences and behaviors, help our company realize personalized marketing, and provide customized recommendations and offers for each consumer”.
Given that so many marketing and sales leaders are curious about what generative AI can do for them, researchers asked what’s holding them back? Responses showed “their top concerns, were the accuracy of generative AI outputs (50%), followed by a range of other potential issues, including employee resistance (39%), ethical considerations (36%), industry compliance (35%), a lack of organizational understanding and norms (33%), the problem of organizational integration 31%), and the exposure of confidential information (28%), and intellectual property rights (23%)”.
Recommendations for the Way Forward
The early adopter position in generative AI provides an opportunity for marketing visionaries to establish an advantage over their competitors by identifying ways their teams can effectively use the technology. One guru of AI marketing says that success will be determined by a “clear understanding, conscious experimentation, and careful consideration of both risks and rewards.” To leverage the potential of new generative AI systems, subject matter experts recommend taking the following steps:
Training
This will enable teams to use and interpret generative AI output and potential capabilities and shortcomings plus recognize when human input is vital.
Employ an intentional trial-and-learn approach
This will help you pinpoint where generative AI could most efficiently improve tasks, content, and overall customer experience.
Establish policies to safeguard proprietary data
Sans widespread generative AI that ensures data protection, treat all inputs and outputs as public domain.
Ensure quality control
Generative AI can and does produce errors and biases. Robust quality-control and oversight mechanisms must be put in place. A brand expert noted that “This vigilance can mitigate potential risks of misinformation or bias, preserving the integrity of both the technology and the brand it serves.”
Enhance (not replace) creativity
Generative AI isn’t limited to an “automater” of tasks. It should become what one expert calls a “capable co-pilot” that helps leaders expand their thinking and develop new ideas. Most experts agree that as its significance increases, its most valuable impacts will come from enhancing, not replacing, human skills — boosting productivity and freeing users for tasks requiring their judgement, emotional intelligence, or other complex decision-making.
Most Common AI Marketing Use Cases Today
AI in marketing is used to make automated decisions based on data collection, data analysis, and additional observations of audience or economic trends that may impact marketing efforts. AI tools use data and customer profiles to learn how to best communicate with customers, then serve them tailored messages at the right time without intervention from marketing team members, ensuring maximum speed and efficiency. AI is used to augment marketing teams or to perform more tactical tasks that require less human interface.
Here are some common AI marketing use cases as of today:
- Data Analysis: AI marketing can help sort through large amounts of data from multiple sources at lightspeed, filtering it down to its essentials and not only analyzing it but also recommending the best elements of future digital marketing campaigns.
- Natural Language Processing (NLP): Creating human-like language for content creation, customer service bots, experience personalization and more.
- Media Buying: Predicting the most effective ad and media placements for a business in order to reach their target audience and maximize marketing strategy ROI.
- Automated Decision-Making: AI marketing tools help a business to decide which marketing or business growth strategy they should use based on past data or outside data inputs.
- Content Generation: Writing both short and long pieces of content for a marketing strategy, such as video captions, email subject lines, web copy, blogs and more.
- Real-time Personalization: Changing an individual customer’s experience with marketing such as a web page, social media, or email to fit the customer’s past preferences to encourage a certain response or action.
- Automated Email Marketing Campaigns: AI tools can help produce more engaging email content by learning about your email list behaviors.
- Forecasting Sales: AI can help marketers understand the predicted outcome of their campaigns and marketing assets. This insight can help marketers develop better campaigns that actually produce sales and ROI.
- Improving Customer Experience: AI can help marketers deliver the best experience for their visitors thus converting them into leads. AI can help increase customer retention and loyalty with personalized content and improve assets.
- SEO Optimization: AI algorithms can analyze website traffic, identify keywords that can help improve search engine rankings, and track competitors’ activity. Plus, using AI-powered tools, marketers can learn about their audience’s preferences and customize their content to match their interests.
Top Level Benefits of AI in Marketing
As we discussed, there are numerous use cases for incorporating AI marketing into a company’s business strategy. The benefits/outcomes are tangible and quantifiable. AI marketing can be an asset in areas such as risk reduction, increased speed, greater customer satisfaction, increased revenue, and more.
Here are a few overarching benefits that can be applied across AI marketing use cases:
- Increased Campaign ROI
- AI platforms can report how to best allocate funds across media channels or analyze the most effective ad placements to engage customers more consistently, thus getting the most value out of campaigns.
- Better Customer Relationships & Real-Time Personalization
- AI marketing can deliver personalized messages to customers at appropriate points (location and device based) and target them with information that will get them to engage or re-engage with the brand.
- Enhanced Marketing Measurement
- AI powered dashboards allow for a more comprehensive view and measurement into what is working so that it can be replicated across channels and budgets allocated accordingly.
- Make Decisions Faster
- AI marketing can use real-time analytics to conduct data analysis more efficiently with faster conclusions based on campaign and customer context to make better media choices.
While AI can be programmed with ethical principles, it lacks the ability to make judgments based on moral values.
What Artificial Intelligence Cannot Do?
For those on the AI bandwagon who feel that AI can do everything, think again. Yes, it is (in many ways) an existential game changer but there are a number of things that artificial intelligence cannot do at a human level. Only by understanding what any tool can and cannot do will its use be as effective as possible. The following is a list to consider:
- Common sense
- Judgement
- Intuition
- Contextual understanding
- Understanding abstract concepts
- Creativity
- Emotions and consciousness
- Tasks involving complex, unstructured data
- Tasks requiring empathy and compassion
- Understanding context
- Tasks that require a lot of experience and intuition
- Tasks that require understanding and interpretation of idiomatic expressions
- Tasks that require common sense understanding of the physical world.
- Explain its decisions
- Interpersonal skills
- Fully replace human workers
- Exercise free will
Let’s briefly discuss them one by one.
- Common sense reasoning:
- This is the ability to use basic knowledge and understanding of the world to make logical inferences and decisions. AI systems currently lack the ability to understand and apply common sense reasoning in the same way that humans can.
- Judgment:
- AI cannot make ethical or moral judgments. AI lacks the ability to consider the broader social, cultural, and ethical implications of its decisions that govern human behavior and decision-making. It involves distinguishing right from wrong and making decisions that align with moral values. While AI can be programmed with ethical principles, it lacks the ability to make judgments based on moral values.
- Intuition and experience
- Intuition involves the ability to make decisions based on gut feelings and instincts and using past experiences and knowledge to make quick and accurate decisions in a given situation. While AI can analyze data and make predictions based on existing and extrapolated data, it lacks the ability to make decisions based on intuition and a deep understanding of a subject that can only be obtained through years of experience.
- Contextual understanding:
- This refers to the ability to understand and interpret information in a way that is influenced by the context in which it is presented. AI systems can struggle with understanding the nuances and subtleties of communication and ambiguities that are influenced by context. AI can misinterpret data, leading to incorrect decisions.
- Understanding abstract concepts:
- This refers to the ability to understand and work with concepts that are not concrete or physically present, such as love, justice, or morality.
- Creativity:
- Creativity is a complex human trait that is influenced by various factors such as emotions, culture, and experiences. It involves thinking outside the box, exploring new ideas, and taking risks. While AI can analyze data, identify patterns and trends, and even generate new ideas based on existing data, it lacks the ability to think outside the box and come up with truly original and creative ideas. AI relies on pre-programmed algorithms that limit its ability to think creatively.
- Emotions and consciousness:
- AI systems are not self-awareness with emotions or consciousness. While AI can recognize and respond to basic emotions such as happiness, sadness, and anger, it lacks the ability to understand complex human emotions such as love, jealousy, and guilt. AI is unable to understand and manage one’s emotions, as well as the emotions of others. It involves being able to empathize with others, provide support, and build relationships.
- Tasks involving complex, unstructured data:
- AI systems can struggle with tasks that involve large amounts of data that is not organized in a structured way. These tasks often require the ability to understand and make sense of data in a way that is similar to how humans would.
- Tasks that require understanding and interpretation of idiomatic expressions:
- Idiomatic expressions are phrases or expressions that cannot be understood by simply understanding the meanings of the words that make them up.
- Exercise free will
- AI can make decisions based on pre-programmed algorithms, but it lacks the ability to make decisions based on personal preferences and values. Free will involves choosing between different options based on one’s desires and beliefs.
The limitations listed above help to underscore that AI cannot replace people. It’s true that AI can do many things exponentially faster than humans. It can also perform data-related tasks that are impossible for the human brain to perform. And, yes, AI’s application in the workplace can result in the elimination of some roles that can be automated, like pure data entry jobs… but what AI cannot replicate are the elements that make humans (for the want of a better word) human.
Jobs R Us
The omnipresent question is not whether AI, and most especially generative AI, is here to stay and will continue growing at an exponential rate. In short, yes, and yes. The question is how it will affect us and how we can prepare for the future. If we look at the data, between January and May 2023, 417,500 jobs were cut but only 3.9k were reportedly because of artificial intelligence — less than 1%. Data aside you still might be wondering how to future-proof your career. The answer is age old. Change always brings the need to re-skill.
Re-skilling is not to be feared. It falls under the heading of professional development, so this might be routine for you. For example, you could explore different AI tools and how to leverage them in your day-to-day role or take a course to learn a new skill. Most experts agree that AI’s most significant impact will likely be helping us be more effective in our careers. But, if your job is impacted, having taken the time to re-skill leaves you more prepared for future opportunities where you present yourself as a multifaceted candidate.
In the process of doing research for this project I ran across an article listing some marketing related jobs that will be impacted (read that improved) but won’t be displaced by AI.
- Chief executives because it’s nearly impossible to automate leadership, emotional intelligence, and personal connections.
- Marketing managers must interpret data, trends, oversee the creation of content and campaigns and nimbly adapt and respond to changes and feedback from the rest of the company and customers.
- Writers need to ideate, truly create, and produce original written material, something that AI writing tools have not yet been able to replicate in the same way as humans.
- Editors have to review writers’ submission for clarity, accuracy, comprehensiveness, and originality.
- Sales managers need high emotional intelligence to hit their monthly quotas, network and collaborate with customers, and motivate and encourage the larger sales team all with the constant need to adapt to new situations.
The best take I found on the issue of job security comes from Kate O’Neill, the author of Tech Humanist and Founder of KO Insights. She explains that jobs that require emotional intelligence will be safer in the immediate future: “This is going to be a continuously moving target, but for the time being, what AI can’t do well is use emotional intelligence, understand situational context, make judgment calls, and generally see nuance and meaning like we do.”
“That means any kind of job that benefits from these kinds of human attributes is better off being done by a human. A computer or robot may assist you in performing efficiently, but for now, you’re the one who adds the expertise on how to perform appropriately,” she added.
Even for jobs more likely to be impacted, there are always reasons why a human with real-life experience and expertise is a better fit. The best way to navigate the fast-moving world of artificial intelligence is to stay informed and adaptable.
Let’s be clear. AI in general, machine learning, and generative AI specifically are here to stay, and the 30,000-foot view is that this is a good thing (with some caveats) and since Pandora’s Box has been opened it will grow exponentially. The key thing to understand is that the AI in all forms that you see today is imperfect and will not be the AI you see in a year and beyond. Flaws aside, it does wonderful things that support and add to the areas we have discussed in this article. Our approach should be as President Ronald Reagan famously told us about foreign policy, “trust but verify”. I will sign off by restating an earlier quote about AI. Treat it as your “co-pilot” with assigned tasks where humans make the final decisions.